It's the use case, stupid: 20-year veteran of Australian analytics, Sri Annaswamy reveals the common thread across two decades of data-led transformation successes and even Gen AI
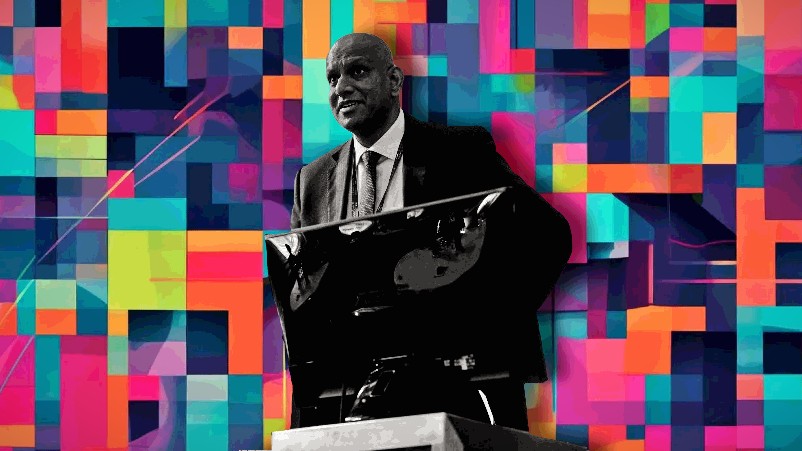
The ways firms use data, and the technology architecture that underpins that usage has changed dramatically over the last 20 years. But one thing has remained constant, according to Sriraman (Sri) Annaswamy, a pioneer of the Australian analytics sector, whose firm Swamy and Associates has been a go-to service provider for some of Australia's biggest organisations in finance and healthcare. Value from each new wave of innovation, whether that be business intelligence, data science, or now, generative AI is realised when it is applied to very specific parts of the business operations.
What you need to know:
- Focus on business unit-specific use cases to realise the greatest value from generative AI, says a pioneer of the Australian data analytics sector with a strong pedigree across some of the country's largest finance and healthcare companies.
- Sri Annaswamy believes the investment Australia's biggest companies have made in their underlying data architectures, and especially in cloud computing, will hold them in good stead as the generative AI era emerges.
- But he warns companies sometimes struggle to keep up operationally with the acceleration of the technology curve.
- And capacity constraints caused by the availability of GPUs in Australia are growing.
- But Annaswamy says investments by data centre providers, and the shift to medium language models and small language models that do specific tasks will help address the issue.
That is a big game changer because I don't need an LLM that has been trained on everything in the encyclopedia to summarise my specific contact centre complaints and queries. I don't need that. But as of today, whatever API version I get still takes me back to that huge LLM model.
As the priorities around data analytics evolved over the last two decades from business intelligence to data science and now to generative AI, the theme that defined success with data has stayed the same, according to Sri Annaswamy, the founder and director of Swamy and Associates, one of Australia's data analytics leaders for the past 20 years.
Annaswamy, who has a reputation for straight and occasionally blunt advice (to customers and journalists alike) told Mi3 Australia, "Ultimately, the value has always been driven when it has been applied to very specific parts of the business operations."
And it's as true today in the emerging era of Generative AI, as it was back at the start of the century when business intelligence was often the driver of analytics investments.
"There's plenty of people using Gen AI for all sorts of things. But the value we are seeing is actually coming from specific parts of the business operation, like contact centres," Annaswamy told Mi3 during a discussion coinciding with his company's 20th anniversary. "For example, helping contact centre agents summarise a customer's complaints, all the problems that customers have had, and then being able to address it. So summarising is a huge impact of generative AI."
Synthetic agents
Another huge impact, Annaswamy believed, is the ability to use generative AI to create synthetic data sets, something he has seen applied by his clients in the finance sector.
"In that case, it was used for fraud. But think about a healthcare company, or think about a health insurance company that wants to deploy AI, but can not get past the data governance rules because there is an enormous amount of private and confidential information. What can they do? You have to use generative AI to create synthetic data sets. Otherwise, you can't use analytics on the scale that you want," he continued. "There's all these foundation technologies, foundational models and everything, but ultimately, only when there are specific business operations and use cases have we seen the value. That's the one common thread."
While the common success factor may have stayed the same, the operating environments have not, however.
"The difference [today] is speed and the scale. Fifteen years ago, when we used to do churn analysis for a bank, at best we had close to maybe a few hundreds of thousands of data points. Banks would typically give us a data set with about 10,000 to 20,000 customers, we would generate actual data sets from them. Now the minimum is like 20 million to 30 million types of parameters that can be used. So the speed and the scale have changed dramatically."
Just as impressive is how quickly results can be generated these days. "Proof of concept times have been cut dramatically," Annaswamy said.
"We are able to get results within a few hours so you don't need a six-month POC to do something. We can do the whole thing in probably six weeks or eight weeks. So POC times have cut down dramatically."
Annaswamy cautioned companies have often struggled to keep pace with the accelerating technology curve. "There is still quite a bit of lag. Customers may not be making decisions at the pace that the service providers would like them to do."
But even here he sees progress.
"Consider things like chatbots, which previously were completely useless because the old natural language program (NLP) chatbots, after two, three queries, couldn't handle anything. Now, because they have generative AI embedded and Gen AI interfaces, they're able to respond much faster and the quality of response is much better. It's still not as good as a human being, but for 90 per cent of queries, you don't need that.
"If you're updating your address, you really don't need to be a human being to do that. You can do that these days with a Gen AI bot and get a good enough response."
Capacity constraints
Annaswamy saw the investments made by Australia's biggest companies in things like cloud computing and data analytics putting them in good stead for the generative AI era. But he also cautioned there are important constraints on uptake in Australia.
"Most of them have either completed or are well progressed in terms of the back-end infrastructure, for instance migrating data into data warehouses or data lakes. All of that is pretty much complete and that's a big part of the journey over the last five to seven years," he said. "Among the ASX 100, if you like, most of them are pretty progressed on the journey, and several of them have completed the journey, so I don't see that as a big hurdle. The big hurdle is the availability of GPUs specifically based in Australia.
"For example, if I want to try something for a healthcare client, and we want to run a healthcare large language model on say, Google. If Google doesn't have enough GPUs dedicated to this market to run these healthcare LLMs, then I can't run them for the client.
"Generally, yes, there's the availability of GPUs, but specifically dedicated to running those applications? It's not very high."
Annaswamy pointed to two things happening in the market that will help address those capacity constraints. "One is that the data centre operators are already building up their capacity. But secondly, and more importantly, over the last three to six months we are already seeing that big move away from using LLMs for everything."
Instead, he said there is now more emphasis on mid language models and small language models.
"That is a big game changer because I don't need an LLM that has been trained on everything in the encyclopedia to summarise my specific contact centre complaints and queries. I don't need that. But as of today, whatever API version I get still takes me back to that huge LLM model."
Small language models have two advantages. The first is they are trained on very specific data sets.
"Let's say you're a healthcare company and you're looking at automating your clinical coding. A small language model will actually be trained on all the clinical coding standards, the DRGs [disease groups], and the various requirements for coding patients' diseases, such as the funding implications. That means a hospital can readily tap into it and use it. Whereas an API created from an LLM is essentially like creating a version of ChatGPT that applies to clinical calling.
"The first one is very specific. I can plug it directly in."
Agentification
That leads to the second advantage. "I don't need that many GPU resources to run it and I don't need a bigger team to run it," Annaswamy said.
"For the second one - the LLM - I need a significant amount of GPU resources, because effectively, I'm running a certain portion of ChatGPT, it has to be in Australia, and I have the pay Chat GPT license fees. That's the difference, and it's called agentification. It is changing things dramatically in favour of small and medium language models, as opposed to these LLMs."